Ready, Set: A.I. Challenges Human Champion at World’s Hardest Game
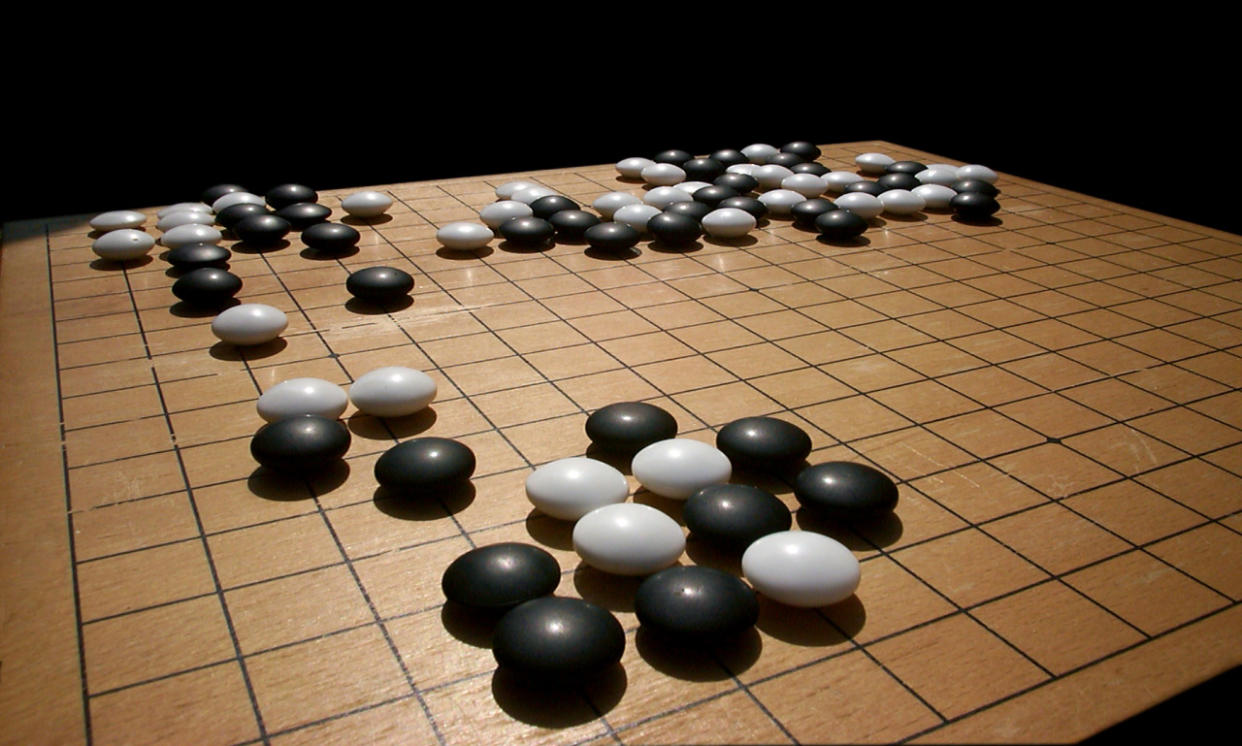
On March 9, humankind will play a very important game.
That’s the day Lee Sedol, world champion Go player, will square off against an artificial intelligence opponent in a series of matches in Seoul, South Korea.
It’s a heavyweight showdown that’s being compared to the famous 1997 matchup between chess champion Garry Kasparov and IBM’s supercomputer Deep Blue. That competition — which Deep Blue won three games to two (with one draw) — was a seismic event in the history of technology. It suggested that supercomputers had surpassed humans in one of our greatest intellectual pursuits.
In many ways, the upcoming Go match is even more significant.
For one thing, Go — the 2,500-year-old Chinese strategy game played with colored stones — is more mathematically complex than chess, by several orders of magnitude. It’s generally considered to be the world’s oldest and most difficult board game.
There’s also the fact that AlphaGo — the name that Google’s DeepMind team has given to its Go-playing supercomputer — essentially taught itself to master the game. Thanks to deep-learning techniques and artificial neural network technology, AlphaGo is a kind of 21st-century digital autodidact. Computer scientists didn’t spend years programming the A.I. with customized algorithms, as with Deep Blue and chess. Instead, AlphaGo programmed itself.

How it works
To learn the game, the AlphaGo system was provided with a database of 30 million moves as played by human experts. That might seem like a lot, but 30 million is actually a minuscule fraction of the total number of moves possible in a game of Go.
After that, the computer played against itself — thousands of times — until the A.I. was able to grasp the game’s higher-level subtleties and dynamics.
“Traditional A.I. methods, which construct a search tree over all possible positions, don’t have a chance in Go,” wrote Google researcher Demis Hassabis, in a blog post accompanying news of the AlphaGo initiative. “So when we set out to crack Go, we took a different approach. We built a system … that combines an advanced tree search with deep neural networks. These neural networks take a description of the Go board as an input and process it through 12 different network layers containing millions of neuron-like connections.”
The breakthrough was significant and surprisingly abrupt. For decades, Go-playing computer programs had been unable to improve themselves past the human amateur level. Professional human players consistently dominated when playing against computers.
AlphaGo arrived on the scene like a supergenius prodigy. When Google matched its system against the other top Go-playing A.I. in a 500-game virtual tournament, AlphaGo went 499-1. Its next bout was even more impressive: Last year, AlphaGo defeated European Go champion Fan Hui 5-0. It was the first time a computer program had ever defeated a professional human Go player.
March’s competition against the world champion is seen as the ultimate test. Sedol is widely considered the best Go player in the world over the past decade. The seven-day match in March will be broadcast live, with a $1 million prize at stake.

Games and evolution
Artificial intelligence has made enormous strides in recent years, largely due to artificial neural-network technology. To radically simplify things, that’s an approach to machine learning in which scientists try to get computers to think like the human brain. Practical applications include computer vision (in which machines learn to see and recognize objects) and speech recognition. You’re able to talk to Siri these days largely due to artificial neural-network technology.
Game theory is another perennially hot area of focus in A.I. research. Teaching computers to win at particular games is often the first step to creating systems that can solve real-world problems.
Arend Hintze, an assistant professor in integrative biology, computer science and engineering at Michigan State University, is working on an A.I. gaming system that incorporates elements of Darwinism. The idea is to create A.I. opponents that evolve on their own as they play human opponents over and over. The A.I. learns as it plays, generating evolved progeny programs, in a kind of super-fast-forward analog of biological evolution.
In terms of game design, the approach has the immediate benefit of making faster and better video games. “The hope is that, instead of having an army of people designing behavior and A.I., those designers can work on story and narrative and the things that make the game more compelling,” Hintze says.
But in the long term, such self-evolving A.I. could have wide-ranging practical applications. As with Google’s AlphaGo program, these superbots can learn on their own and overcome challenges outside the original purview of the task.
“Machines that evolve are much better able to deal with a plethora of problems, rather than the one task that they were created for,” Hintze says. “We know that evolution is the thing that resulted in human intelligence. So why not use this to make intelligent machines?”

Poker faces
Meanwhile, over at Carnegie Mellon University, Professor Tuomas Sandholm is working on A.I. systems in the context of another enormously complex game: poker. Over the last several years, Sandholm and his team have designed a poker-playing supercomputer program known as Claudico. At a two-week tournament held last year, Claudico demonstrated that it could hold its own against professional poker players in the popular poker variant No Limit Texas Hold ’em.
Sandholm is one of the world’s leading experts on A.I. and game theory, and he says he’s very much looking forward to seeing the results of AlphaGo’s match against Sedol. “The Deep Mind people have put deep-learning techniques together with existing algorithms for Go to create what is, I think, the world’s strongest program for Go,” he says.
But for Sandholm, poker represents an entirely different kind of challenge. Games like chess and Go are known as complete information games, Sandholm says. “When it’s my turn to go, I know everything. There is no hidden information,” he explains.
Poker, on the other hand, is an imperfect information game. Players do not know the value of the cards their opponents are holding or of the face-down community cards. That introduces strategy elements like aggressive wagering and bluffing, and these present a whole different set of challenges for A.I.
“These imperfect information games are much more important for real-world applications,” Sandholm says. “Think about negotiations, or bidding at auctions, or cybersecurity applications. It’s very important for sophisticated A.I. to know what to do when the other agents that the software is interacting with are trying to deceive.”
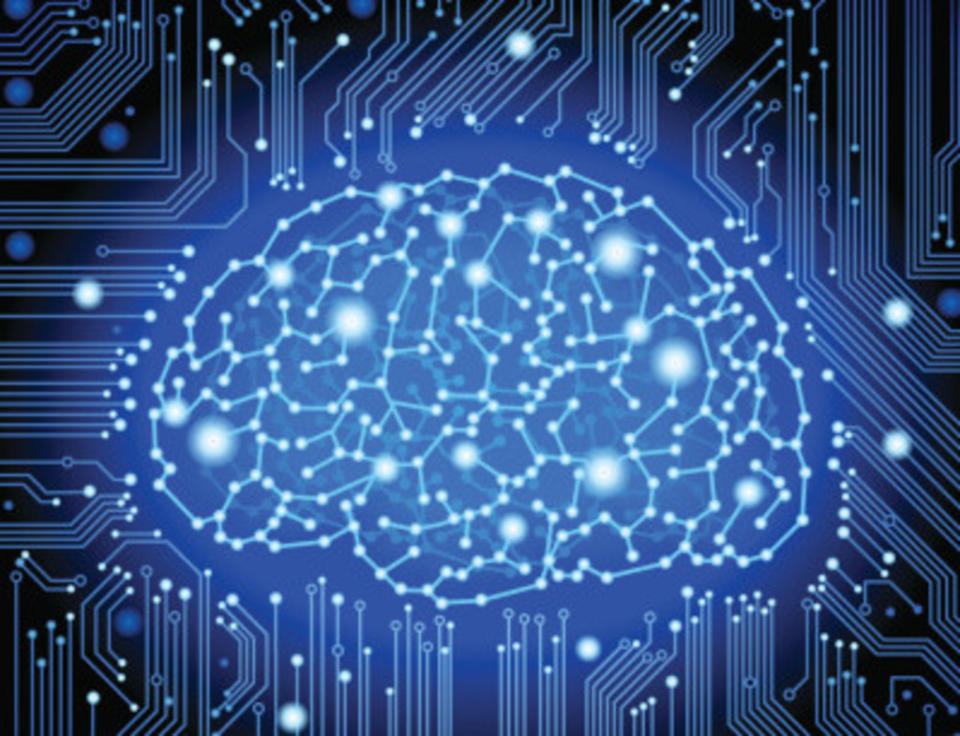
Playing for keeps
AlphaGo’s big match in March will be closely followed by pretty much everyone in the field of A.I. It could prove to be a watershed moment, like Deep Blue versus Kasparov. But no matter what the result, it’s clear that things have changed drastically in the nineteen years since that historic match.
A.I. is learning to play all kinds of games, and thanks to neural network technology, in many cases it’s teaching itself. The potential downside of all this is a familiar trope in scary science fiction stories. But researchers like Sandholm prefer to look at the upside of teaching machines how to play games.
“For instance, we’ve done [simulated] medical treatment planning, where the A.I. treats the disease as an opponent in a game,” he says. “Each game is customized to the disease at hand, whether it’s diabetes or cancer.”
If A.I. can rack up some victories in those kinds of games, everybody wins.